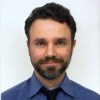
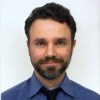
The goal of autonomous driving systems (ADS) research is to give safer and economical mobility options. However, the operational design domains (ODD) of state-of-the-art ADS constraints the deployment under sunny to cloudy conditions. The weather phenomena impair the perception and navigation capabilities of ADS, so their availability is limited by geography and seasons. Furthermore, unstable vehicle control induces a strong feeling of risk to the passengers. For ADS to continue moving forward to the next era, research efforts are necessary to enable autonomous cars to get past all the…
Neuromorphic computers are emerging computing systems that operate on the principles of the central nervous system. They implement neurons and synapses in hardware, supporting biology-inspired synaptic plasticity. These systems can perform several different types of scientific computations with significantly lower energy footprints compared to a conventional CPU-based computer. Future high-performance neuromorphic computers are expected to aggregate multiple heterogeneous neuromorphic hardware nodes to solve…
Machine learning on tiny IoT devices based on microcontroller units (MCU) is appealing but challenging: the memory of microcontrollers is 2-3 orders of magnitude smaller even than mobile phones. I’ll present the MCUNet project, a framework that jointly designs the efficient neural architecture (TinyNAS) and the lightweight inference engine (TinyEngine), enabling ImageNet-scale inference on microcontrollers. Beyond inference, our…
Uncrewed aerial vehicles (UAVs), or drones, are increasingly being used to deliver goods from vendors to customers. To safely conduct these operations at scale, drones are required to broadcast position information as codified in remote identification (remote ID) regulations. However, location broadcast of package delivery drones introduces a privacy risk for customers using these delivery services: Third-party observers may leverage broadcast drone trajectories to link customers with their purchases, potentially resulting in a wide range of privacy risks.
We propose a…
Machine learning models are increasingly being incorporated into real-world systems, targeting domains such as robotics, healthcare, and software systems. A key challenge is ensuring that such systems are trustworthy. I will describe two strategies for verifying correctness properties for such systems. The first strategy leverages ideas from statistical verification to provide provable correctness guarantees. In particular, we show how to quantify the uncertainty of any given model in a way that satisfies PAC correctness guarantees, and then leverage these uncertainties to ensure safety.…