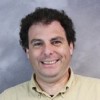
Compared to typical single-agent decision problems, general sum games offer a panoply of strategies for maximizing utiity. In many games, such as the well-known Prisoner's dilemma, agents must work together, bearing some individual risk, to arrive at mutually beneficial outcomes. In this talk, I will discuss several algorithmic approaches that have been developed to identify cooperative strategies in non-cooperative games. I will describe an analysis of value-function-based reinforcement learning, the pivotal role of assumptions about the opponent, the computational benefits of side payments, a multi-stage extension of the computational folk theorem, and the role of cognitive hierarchies.
Michael L. Littman's research in machine learning examines algorithms for decision making under uncertainty. He has earned multiple awards for teaching and his research has been recognized with three best-paper awards on the topics of meta-learning for computer crossword solving, complexity analysis of planning under uncertainty, and algorithms for efficient reinforcement learning. Littman has served on the editorial boards for the Journal of Machine Learning Research and the Journal of Artificial Intelligence Research. He was general chair of International Conference on Machine Learning 2013 and program chair of the Association for the Advancement of Artificial Intelligence Conference 2013.