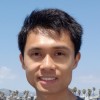
Inspired by the potential of artificial intelligence (AI) to improve access to expert-level medical image interpretation, several organizations began developing deep learning-based AI systems for detecting diabetic retinopathy (DR) from retinal fundus images around 2015. Today, these AI-based tools are finally being deployed at scale in certain parts of the world, often bringing DR screening to a population lacking easy access to timely diagnosis. The path to translating AI research into a useful clinical tool has gone through several unforeseen challenges along the way. In this talk, we share some lessons contrasting a priori expectations (“myths”) with synthesized learnings of what truly transpired (“reality”), to help others who wish to develop and deploy similar medical AI tools. As illustrative examples of challenges and corresponding solutions, I will cover case studies in deep learning for diabetic retinopathy, glaucoma, and more. Finally, I will briefly touch on explainability and an exciting paradigm of "learning from deep learning" where instead of people labeling data to train models, models learn from data and people derive new insights from these models.
Yun Liu is a staff research scientist in Google Health. In this role he focuses on developing and validating machine learning for medical imaging across multiple fields: pathology, ophthalmology, radiology, and dermatology. Yun completed his PhD at Harvard-MIT Health Sciences and Technology, where he worked on predictive risk modeling using biomedical signals, medical text, and billing codes. He has previously also worked on predictive modeling for nucleic acid sequences and protein structures. Yun completed a B.S. in Molecular and Cellular Biology and Computer Science at Johns Hopkins University.