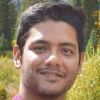
Neural networks have become ubiquitous when it comes to learning enabled cyber-physical systems, like autonomous cars and closed loop medical devices. Such safety critical applications, lead to a compelling use case for formal verification approaches. In this talk I will be presenting an approach for verification of neural network controllers for closed loop dynamical systems. Given a neural network and a set of possible inputs to the network described by polyhedral constraints, the aim would be to compute a safe over-approximation of the set of possible output values. I would present an efficient range estimation algorithm that iterates between an expensive global combinatorial search using mixed-integer linear programming problems, and a relatively inexpensive local optimization that repeatedly seeks a local optimum of the function represented by the network. Next, we show how this can be combined with tools like Flow*, to compute reach sets for systems where the dynamics are modeled as an ODE.
I am Souradeep Dutta, a 4th year PhD candidate at the Dept of Electrical Computer and Energy Engineering, University of Colorado at Boulder. I am a part of the CUPLV research group, where my adviser is Sriram Sankaranarayanan.