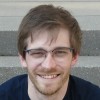
For safety, AI systems in health undergo thorough evaluations before deployment, validating their predictions against a ground truth that is assumed certain. However, this is actually not the case and the ground truth may be uncertain. Unfortunately, this is largely ignored in standard evaluation of AI models but can have severe consequences such as overestimating the future performance. To avoid this, we measure the effects of ground truth uncertainty, which we assume decomposes into two main components: annotation uncertainty which stems from the lack of reliable annotations, and inherent uncertainty due to limited observational information. This ground truth uncertainty is ignored when estimating the ground truth by deterministically aggregating annotations, e.g., by majority voting or averaging. In contrast, we propose a framework where aggregation is done using a statistical model. Specifically, we frame aggregation of annotations as posterior inference of so-called plausibilities, representing distributions over classes in a classification setting, subject to a hyper-parameter encoding annotator reliability. Based on this model, we propose a metric for measuring annotation uncertainty and provide uncertainty-adjusted metrics for performance evaluation. We present a case study applying our framework to skin condition classification from images where annotations are provided in the form of differential diagnoses. The deterministic adjudication process called inverse rank normalization (IRN) from previous work ignores ground truth uncertainty in evaluation. Instead, we present two alternative statistical models: a probabilistic version of IRN and a Plackett-Luce-based model. We find that a large portion of the dataset exhibits significant ground truth uncertainty and standard IRN-based evaluation severely over-estimates performance without providing uncertainty estimates.
Links: https://arxiv.org/abs/2307.09302 https://arxiv.org/abs/2307.02191
David is a research scientist at Google DeepMind interested in robust and safe deep learning. Before, he completed his PhD at the Max Planck Institute for Informatics which included an internship at Google DeepMind and a collaboration with IBM Research. His PhD was supported by a Qualcomm Innovation Fellowship 2019 and received the DAGM MVTec Dissertation Award 2023. Other notable honors include an outstanding paper award at the CVPR 2021 CV-AML workshop, participation in the 7th and 10th Heidelberg Laureate forum, the RWTH Aachen University Springorum Denkmünze as well as the STEM-Award IT 2018 for his master thesis, and several national scholarships. He was repeatedly recognized as an outstanding/top reviewer for CVPR, ICML and NeurIPS.