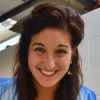
Artificial Pancreas (AP) systems refer to a set of increasingly closed-loop biomedical devices which seek to automate blood glucose regulation in individuals with type-1 diabetes mellitus (T1DM). While incredibly promising for the treatment of T1DM, these systems present a one-sided control problem, able to dose insulin to decrease blood glucose levels, but unable to counteract the effects of too high a dose. Additionally, safety is critical: too high a dose leading to long-term organ damage, coma, and potentially death to an individual. Thus, the need to test and formally guarantee the safety of such systems is critical. In order to test such systems prior to patient trials, in-silico modeling of patient physiology along with the closed-loop interactions between the control algorithms and human physiology is utilized. Historically, nonlinear ordinary differential equations (ODE) models have been used as the patient models. However, such models are deterministic and general, which has resulted in a mismatch between testing for general use of AP systems, and the translation of a system to a specific individual. In this work, we present two types of nondeterministic models which we have developed to model human glucose-insulin physiology on a patient-specific level: linear ARMAX models and neural networks. We demonstrate how these models are learned from data AP systems are already collecting, requiring no additional intrusive measurements, and present three methods for quantifying uncertainty/error in the models which can lead to more exhaustive verification both in model conformance and closed-loop safety verification. Finally, we utilize these models within two tasks (1) performing analysis and patient-specific tuning of a PID control system from literature, and (2) real-time learning of a model predictive control system with safety guarantees, with results shown for both in-silico and real patient data.
Taisa Kushner is a PhD candidate in the BioFrontier's Institute Interdisciplinary Quantitative Biology program, and Department of Computer Science at the University of Colorado Boulder. She is advised by Dr. Sriram Sankaranarayanan in the Programming Languages and Verification Lab, with research focus on data-driven modeling and control verification for artificial pancreas systems. Taisa currently consults for Tandem Diabetes Care and has previously held positions at Microsoft Research, the University of Minnesota Twin Cities, and the Center for Diabetes Technology at the University of Virginia. She holds a B.A in Mathematics and Fine Art from St. Olaf College in Northfield, MN, and M.S in Applied Mathematics from the University of Colorado Boulder.