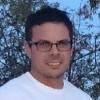
Anomaly detection is the process of identifying abnormal events. The proper identification of anomalies can be helpful for almost any domain and is critical in achieving level 4 and 5 autonomy for self-driving cars. However, classical anomaly detection is principally rooted in point-based anomalies, those anomalies that occur at a specific point, which are only a small subset of the more general range-based anomalies that occur in real-world systems.
In this talk, we will discuss necessary advances in anomaly detection to make it relevant in today’s range-based systems. We will also briefly discuss peripheral issues related to anomaly detection, such as neural network verification and the importance of training data.
Justin Gottschlich is a senior staff research scientist at Intel Labs. He is the Intel principal investigator and co-founder of the joint Intel-NSF CAPA research center for programmability of heterogeneous systems. His current research interests focus on machine learning with an emphasis on software generation, anomaly detection, and autonomous systems. Justin currently oversees academic collaborations at Brown and Stanford Universities and an industrial collaboration between Intel and BMW for anomaly detection on autonomous vehicles.
Justin is a founding member of the Machine Learning and Programming Languages (MAPL) workshop. He has been the general and program chairs for MAPL and TRANSACT and the vice-chair of the C++ Standards Transactional Memory Working Group (SG5). Justin has over two dozen publications and holds a dozen patents with over forty pending. He completed his PhD at the University of Colorado – Boulder and is the CEO of Nodeka, LLC, an online gaming company that he has run for 20 years.