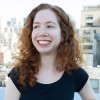
The collection of massive observational datasets has led to unprecedented opportunities for causal inference, such as using electronic health records to identify risk factors for disease. However, our ability to understand these complex data sets has not grown the same pace as our ability to collect them. Instead causal inference has mainly focused on identifying pairwise relationships between variables. However, when the inference output is a large network of relationships, it can be difficult for non-experts to make sense of, and can lead to ineffective actions. This talk discusses recent methods developed for automatically extracting complex relationships from data and how we can make better use of causal information for decision-making.
Samantha Kleinberg is an Assistant Professor of Computer Science at Stevens Institute of Technology. She received her PhD in Computer Science from New York University in 2010 and was a Computing Innovation Fellow at Columbia University in the Department of Biomedical informatics from 2010-2012. She is the recipient of NSF CAREER and JSMF Complex Systems Scholar Awards and is a 2016 Kavli Fellow of the National Academy of Sciences. She is the author of Causality, Probability, and Time (Cambridge University Press, 2012) and Why: A Guide to Finding and Using Causes (O’Reilly Media, 2015).